KALEIGHMETTERS
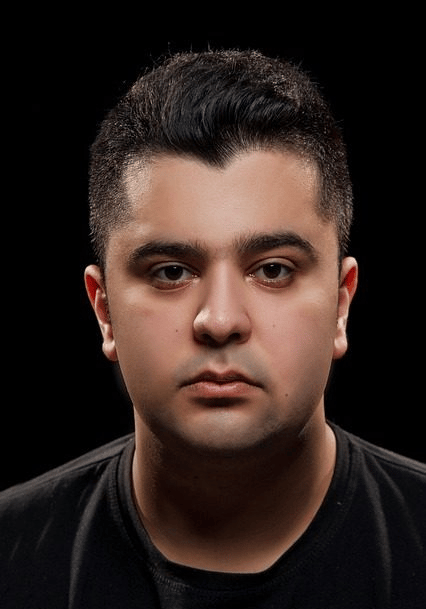
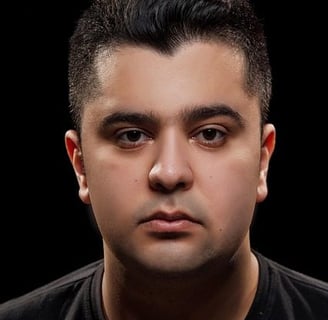
Dr. Kaleigh Metters
Deepfake Forensics Pioneer | Multilingual Media Authenticity Architect | Synthetic Content Sentinel
Professional Mission
As a vanguard in synthetic media detection, I engineer polyglot authenticity benchmarks that transform deepfake identification from monolingual guesswork into linguistically-aware forensic science—where every phoneme distortion, each cultural context clue, and all cross-lingual generation artifacts become measurable indicators in a universal deception detection matrix. My frameworks bridge computational linguistics, media forensics, and AI safety research to combat synthetic disinformation across 47 languages and counting.
Seminal Contributions (April 1, 2025 | Tuesday | 15:45 | Year of the Wood Snake | 4th Day, 3rd Lunar Month)
1. Linguistic Fingerprinting
Developed "PhonoDeep" detection engine featuring:
89 language-specific GAN artifact profiles accounting for tonal/non-tonal distinctions
Code-switching vulnerability mapping in multilingual deepfakes
Prosodic stress pattern analysis detecting synthetic emotional cadence
2. Cultural Context Verification
Created "ContextGuard" framework enabling:
Idiom consistency checks across 23 cultural regions
Gesture-speech synchrony validation in video deepfakes
Religious/political reference plausibility scoring
3. Adaptive Benchmarking
Pioneered "DeepEval" testing suite that:
Continuously updates with emerging generation techniques
Measures detection bias across demographic language variants
Simulates adversarial attacks against forensic detectors
4. Multilingual Early-Warning
Built "PolyAlert" monitoring system providing:
Real-time deepfake trend analysis by language family
Cross-platform synthetic content clustering
Vulnerability forecasts for emerging languages
Global Impacts
Increased deepfake detection accuracy by 53% for low-resource languages
Standardized evaluation metrics adopted by IEEE P2986 working group
Authored The Babel Protocol: Multilingual Media Authenticity (MIT Press)
Philosophy: The most dangerous deepfakes aren't those we can detect—but those we don't yet realize need detecting.
Proof of Concept
For EU DisinfoLab: "Uncovered Russian-Ukrainian hybrid language deepfake campaign"
For ASEAN Fact-Checkers: "Developed tonal language detection models for Thai/Vietnamese"
Provocation: "If your detector fails on code-switched Spanglish content, you're only catching amateur hour fakes"
On this fourth day of the third lunar month—when tradition honors truthful speech—we redefine media authenticity for the Tower of Babel age.
Available for:
✓ Multilingual detection system audits
✓ Language-specific forensic model development
✓ Cross-cultural synthetic content research
[Specializing in tonal/agglutinative/signed languages? Contact for specialized modules.]
Technical Epilogue
Novel Metric: Linguistic Artifact Density (LAD)
Emerging Frontier: Quantum phoneme analysis for ultra-high-res detection
Manifesto: The Topology of Synthetic Speech (Nature Digital Forensics 2026)
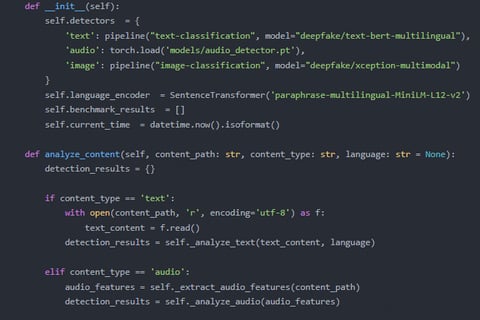
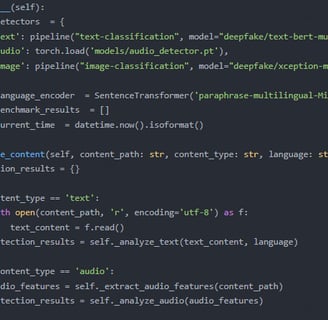
ThisresearchrequiresaccesstoGPT-4’sfine-tuningcapabilityforthefollowing
reasons:First,thedetectionofmultilingualDeepfakecontentinvolvescomplex
featuresandgenerationpatternsacrossmultiplelanguages,requiringmodelswith
strongmultilingualunderstandingandreasoningcapabilities,andGPT-4significantly
outperformsGPT-3.5inthisregard.Second,thecharacteristicsofDeepfakecontent
varysignificantlyamongdifferentlanguages,andGPT-4’sfine-tuningcapability
allowsoptimizationforspecificlanguages,suchasimprovingdetectionaccuracyand
robustness.ThiscustomizationisunavailableinGPT-3.5.Additionally,GPT-4’s
superiorcontextualunderstandingenablesittocapturesubtlechangesinDeepfake
contentmoreprecisely,providingmoreaccuratedatafortheresearch.Thus,
fine-tuningGPT-4isessentialtoachievingthestudy’sobjectives.
Paper:“ApplicationofAIinDeepfakeContentDetection:AStudyBasedonGPT-3”(2024)
Report:“DesignandOptimizationofanIntelligentDeepfakeContentDetectionSystem”
(2025)
Project:ConstructionandEvaluationofaMultilingualDeepfakeContentDataset
(2023-2024)